Mu Hua is a post-graduate student at the University of Lincoln and working on ULTRACEPT’s work package 1.
University of Lincoln researcher Mu Hua attended and presented at the International Joint Conference on Neural Networks 2021 (IJCNN 2021) which was held from 18th to 22nd July 2021. Although originally scheduled to be held in Shenzhen, China, due to the ongoing international travel disruption caused by Covid-19, the conference was moved online.
IJCNN 2021 is the flagship annual conference of the International Neural Network Society (INNS) – the premiere organisation for individuals interested in a theoretical and computational understanding of the brain and applying that knowledge to develop new and more effective forms of machine intelligence. INNS was formed in 1987 by the leading scientists in the Artificial Neural Networks (ANN) field. The conference promotes all aspects of neural networks theory, analysis and applications.
This year IJCNN received 1183 papers submitted from over 77 different countries. Of these, 1183 papers, 59.3% were accepted. All of them are included in the program as virtual oral presentations. The top ten countries where the submitting authors come from are (in descending order): China, United Sates, India, Brazil, Australia, United Kingdom, Germany, Japan, Italy, Brazil, Japan, Italy and France. The event was attended by more than 1166 participants and featured special sessions, plenary talks, competitions, tutorials, and workshops.
Representing the University of Lincoln, Mu Hua presented his paper Mu Hua, Qinbing Fu, Wenting Duan, Shigang Yue “Investigating Refractoriness in Collision Perception Neural Network”, (IJCNN 2021) with a poster demonstrating that numerical modelling refractory period, a common neuronal phenomenon, can a promising way to enhance the stability of currently LGMD neural network for collision perception.
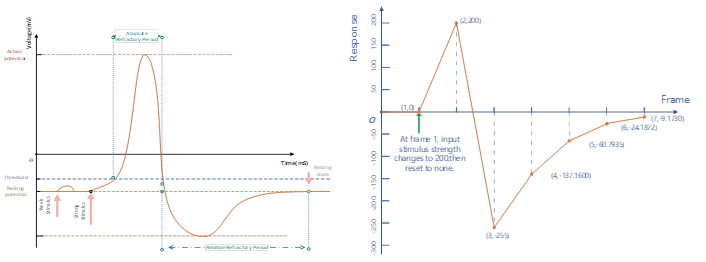
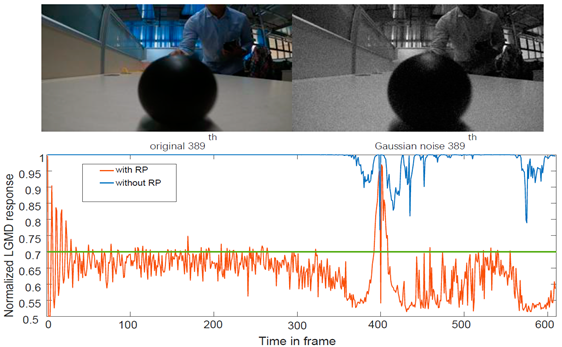
Abstract
Currently, collision detection methods based on visual cues are still challenged by several factors including ultra-fast approaching velocity and noisy signal. Taking inspiration from nature, though the computational models of lobula giant movement detectors (LGMDs) in locust’s visual pathways have demonstrated positive impacts on addressing these problems, there remains potential for improvement. In this paper, we propose a novel method mimicking neuronal refractoriness, i.e. the refractory period (RP), and further investigate its functionality and efficacy in the classic LGMD neural network model for collision perception. Compared with previous works, the two phases constructing RP, namely the absolute refractory period (ARP) and relative refractory period (RRP) are computationally implemented through a ‘link (L) layer’ located between the photoreceptor and the excitation layers to realise the dynamic characteristic of RP in discrete time domain. The L layer, consisting of local time-varying thresholds, represents a sort of mechanism that allows photoreceptors to be activated individually and selectively by comparing the intensity of each photoreceptor to its corresponding local threshold established by its last output. More specifically, while the local threshold can merely be augmented by larger output, it shrinks exponentially over time. Our experimental outcomes show that, to some extent, the investigated mechanism not only enhances the LGMD model in terms of reliability and stability when faced with ultra-fast approaching objects, but also improves its performance against visual stimuli polluted by Gaussian or Salt-Pepper noise. This research demonstrates the modelling of refractoriness is effective in collision perception neuronal models, and promising to address the aforementioned collision detection challenges.
This paper can be freely accessed on the University of Lincoln Institutional Repository Eprints.